Suzanne Lane: Lufkin Award Winner Interview
Published on April 7, 2025
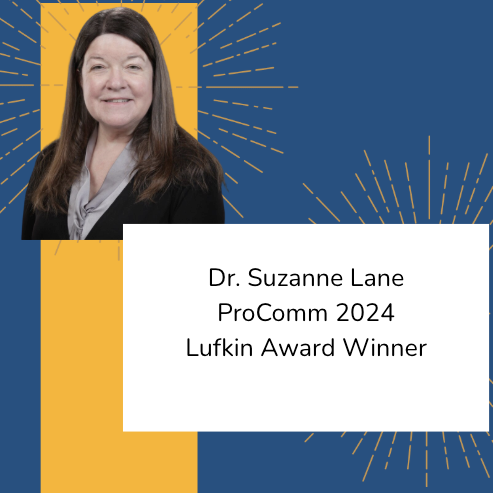
Dr. Lane won the James M. Lufkin Award for Best IPCC/ProComm Paper, which highlights papers submitted to the annual conference. Her work, titled “Meeting ABET Outcomes through Teaching Students to Analyze the Ethics of an AI System,” can be found in the IEEE ProComm 2024 Proceedings in IEEE Xplore.
I was able to chat with my colleague, Dr. Suzanne Lane, on a nice day in March 2025. The interview notes below were transcribed from that online meeting. ~Traci Nathans-Kelly
***
Traci Nathans-Kelly (TNK): Why did you choose this topic or what was the draw for exploring this issue?
Suzanne Lane (SL): In the summer of 2023, I was putting together my syllabus for Engineering Communications and really thinking about what would be a good team project for those undergrad engineers since part of the course addresses team collaboration and communication. I had just moved to Cornell, and for the past 15 years I was at MIT, where I was teaching communication embedded in actual engineering classes where the projects were stemming from their engineering work.
So, I was thinking about what would make a good project for a freestanding class with engineers from all different disciplines, one that would be engineering-focused, but that wouldn’t require any discipline-specific technical skills or lab equipment. And I happened to be a representative at IEEE Sections Congress that summer, and they were giving a presentation on AI standards, which was relatively new at that time. They spoke about how IEEE, which creates standards across the electrical and electronics industries, wanted to have an AI testing element that looked at a lot of the different concerns that people are very familiar with in terms of AI: intellectual property, privacy, security, fairness, bias, sustainability, et cetera. The idea was to have an IEEE “seal of approval,” which may not have been the exact term that they used, that had been vetted by IEEE according to particular standards.
As I was listening to that presentation, I realized that assessing the ethics of an AI system was something that our students could do and maybe certainly not at the level and not as intensely as IEEE was doing it, but that they could choose specific elements of privacy or bias, et cetera, choose one specific AI system and one use case for it, and work in teams to develop a testing methodology to analyze the system. The students would have to propose this work and justify all of their choices, and they would have to give a final presentation.
Clearly within an engineering communication class, this was a project that involved them in some really central issues in engineering at the moment. Whether these students are computer scientists or electrical engineers or some other type of engineer, they are probably going to have to choose whether to adopt or incorporate particular AI technologies into their work. I thought that having a robust understanding of all the issues that you should take into consideration as you make that decision would be a really good experience for them. The course provides such a great opportunity for them to work through these ethical issues in practice. That said, IEEE ProComm was obviously the site where I wanted to then publish the results of this work since IEEE was where I originally came up with the idea for doing this project in my class.
TNK: I didn’t know that that’s where the seed of that idea was! And, moving forward then, where do you see this project going next?
SL: I want to contextualize that answer with where my research has been and what my main interests are. Within engineering communication, my interests are really in engineering reasoning and genres, especially either new genres in engineering or the ways that engineering genres are changing due to changes in disciplinary reasoning and in new media. For instance, I’m interested in the ways that research articles have undergone a really rapid shift in the last 20 years because they’re now mostly published online. Consequently, the page limits are not the same. And you can use color, and you can have all kinds of supplementary materials and links and so on. I find these questions of the intersection of rhetorical theory and then engineering discipline, really, really interesting.
The other area of my research historically has been pedagogy and pedagogical tools. I’m engaged with two things, in particular, in terms of thinking about AI; the first one is thinking really deeply rhetorically about the output of large language models and how that functions and the ways it is similar to, but also distinct in very important rhetorical ways from human communication. And I feel like that is only beginning to be thought about. The second one is thinking about the reasoning processes in creating AI because so much of it is machine learning—these deep learning techniques are not fully understood in themselves, even by the people using them. And there’s a lack of transparency around how we are getting the outputs that we’re getting. We understand it at high level, but we don’t necessarily understand all the selections. Thinking about a whole discipline reasoning with these sets of black boxes is just really, really fascinating to me.
TNK: How will you move forward with this? What is your next step forward?
SL: I think for both, the next step is to narrow all the questions in order to make them manageable, and then identify an appropriate corpus and start analyzing. In my previous work, one of the things I’ve done is what are called reasoning diagrams. In that project, which has been incredibly fruitful, my research partners and I have created about half a dozen reasoning diagrams, which are a kind of knowledge map that maps disciplinary reasoning but also the rhetorical issues involved in communicating that disciplinary reasoning.
For instance, by reading many articles in a field and talking to experts in that field, we found the main conceptual categories that field thinks with. As an example for material science, materials is obviously a main concept, so is processing, and the properties of materials, et cetera. Some disciplines have codified their central conceptual categories more than other disciplines have. We started with materials science, and it turns out we were very lucky too, because materials science had relatively recently codified what they called the materials science tetrahedron, which was the relationship between their central concepts or concept categories.
As it turns out, within disciplines researchers follow a pretty patterned way of connecting those concept categories, and each connection addresses a particular kind of question, or what we would call a “stasis” in rhetorical theory. So as an example, going back to materials science, the question of whether we have a particular material in a specific context is in the stasis of fact, it’s either there in that context or it’s not there in the context. The question of whether that material has particular properties is also a question of fact. You measure the properties; your measurement might not align with my measurement, but to the extent that we might argue about the properties, we would be arguing about facts. But whether those properties are useful in that context is a question of value or evaluation. Properties have no inherent value in themselves. They’re only of value for a particular purpose and context. Stasis theory really helps us then understand how you follow a reasoning pathway through particular kinds of questions between these central concept categories in a field.
I would really love to do a genre analysis of reasoning in AI, and I think that AI is varied enough that it probably requires multiple different analyses with sub-disciplines, like large language models are different from just deep learning decision algorithms. I’d want to come to an understanding of the subcategories within AI, probably starting with large language models because that is what’s producing the output that people are taking as rhetorical. But I’d also like to get a deeper understanding of the academic discourse and reasoning within AI.
Finally, I would be very interested in thinking as a third project, sort of doing a really deep analysis of the rhetoric around AI and how people come to understand what AI is and what it’s standing for in our culture at the moment. But all of these projects would require essentially a similar approach, which is narrowing the question to something more specific, identifying a corpus, and then analyzing texts.
TNK: All of those projects sound amazing to me. I want to switch gears just a bit and ask this: What advice might you give someone considering their doctorate or research in similar topics?
SL: The more I teach students to do project work, which is relevant to this paper as well, the more I put time into helping them understand how to scope a project, because there are some systematic ways of doing it, but there’s a little bit of an art to it as well, I think. We often start with big, amorphous ideas for projects, and to make them workable, we need to define, refine, and narrow their scope. So, you start with an interesting question, but then essentially what you start doing is trying to find all the things that you can rule out about that question. When scoping, you are trying to control the variables as much as possible, but you want to end up with well-designed student projects as test cases. I often think of narrowing the scope in terms of a process I learned in my undergraduate major in chemical engineering, which was to identify your boundary conditions before solving a problem.
TNK: Any last notes for us?
SL: I guess the one thing that I would say, there’s a way in which–because my paper is about teaching and it’s about a very specific project in a very specific context–that people may see it only in that narrow box.
Instead, I would like people to think about the ways in which my paper not only explains what the students have to do in this specific project, but also the instruction about AI and ethics that I provide for them. There are a lot of really important concepts about AI and examples in my paper that could be extracted from that specific context and applied elsewhere. My paper, for instance, has a good introduction to the Eliza effect, which is our human propensity to ascribe to AI human-like features and to talk about it as if the AI is thinking about its output, for instance, which obviously it’s not doing.
Overall, I hope the paper contributes to the growing conversation about how we teach students about interacting with and communicating about AI.
IEEE ProComm thanks Dr. Lane for her time for this interview.